SysMedOs
Integration von Oxidativen Stress in eine systemmedizinische Betrachtung von Fettleibigkeit und deren Komplikationen
Vorhabenbeschreibung:
Das Ziel des Projektes ist es, ein umfangreiches Wissen im Bezug auf die Verhaltensweise unterschiedlich oxidierter Versionen von Lipiden und Proteinen für die Fettleibigkeit und deren Komplikationen unter Verwendung systemmedizinischer integrierter Betrachtungsweisen zu erlangen. Das Projekt kombiniert klinische Parameter und Omics-Daten für oxidierte Lipide und lipid-modifizierte Proteine sowie öffentlich zugängliche Omics-Literaturdaten. Dies ermöglicht eine mathematische Modellierung der Auswirkung von Oxidativem Stress in Fettgewebe zur Unterscheidung zwischen metabolisch gesunden Individuen von Subjekten mit hohem metabolischem Risiko. Durch die Verwendung von integrierten quantitativen Daten der durchgeführten Oxlipidomics und Proteomics der LPP-modifizierten Proteine (Lipox-proteomics; für Fettgewebe und Plasmaproben) in Kombination mit den zugänglichen Literaturdaten und dem daraus abgeleiteten mathematischen Modell, verspricht diese Herangehensweise eine informative und tiefgründige Betrachtung zum Verständnis der Fettleibigkeit und deren Komplikationen.
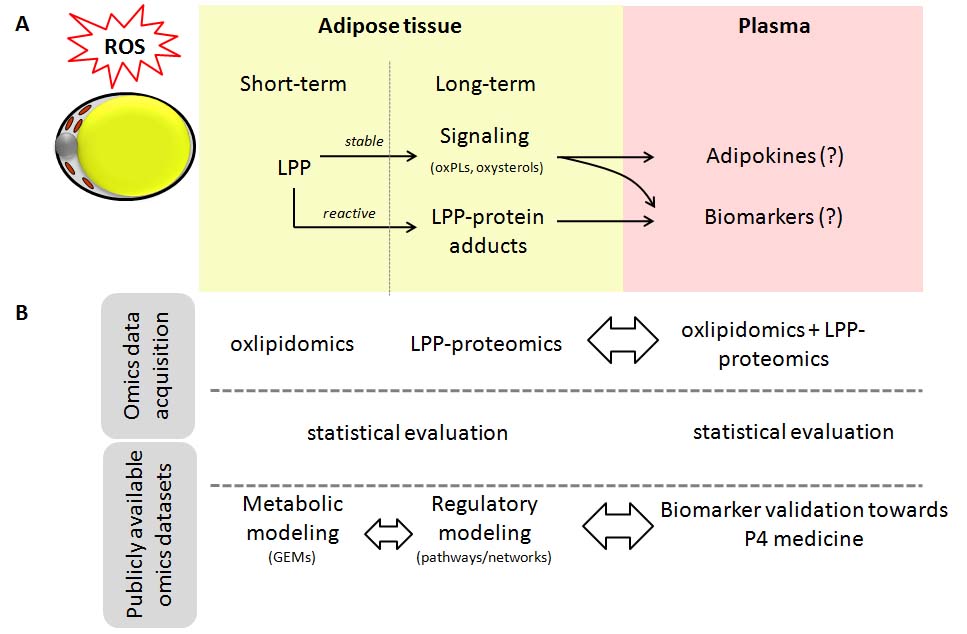
Arbeitsplan:
Das Projekt beinhaltet eine Kombination aus vielen Herangehensweisen wie der Erstellung von Omics-Daten, klinischer Charakterisierung, statistische Evaluierung und mathematische Modellierung. Die Verwendung von bisher publizierten und zugänglichen Omics-Daten sowie mathematischen Modellen des Fettgewebes als Gerüst resultiert in einer Generierung neuer Daten der oxidierten Lipide und Proteine, die mittels genome-scale metabolic modeling und reulatory protein networks analysis integriert werden. Dies ermöglicht eine systemmedizinische Betrachtung metabolisch gesunder und erkrankter Fettleibigkeits-Phenotypen und kann für die Identifizierung von potentiellen Biomarkern und therapeutischen Zielen genutzt werden. Diese Strategie wird weiterführend in Blutplasmaproben fettleibiger Individuen und schlanken Subjekten validiert werden.
Zusammenfassung über das Krankheitsgebiet:
Die Ergebnisse des Projektes besitzen einen sehr hohen diagnostischen, prognostischen und therapeutischen Wert, da zum einen die signifikanten Merkmale und Auswirkungen der Fettleibigkeit adressiert werden. Zum anderen unterstützen die Resultate die Suche nach neuen Biomarkern und die Entwicklung neuer Interventionstherapien sowie diagnostische/prognostische Assays. Diese verlässlichen Biomarker werden die klinische Entwicklung vorantreiben, da sie (i) die Beobachtung des Krankheitsfortschritts und die Therapie-Evaluierungen beschleunigen, (ii) Informationen über Drug-Target-Interaktionen, allgemeine Änderungen der krankheitsspezifischen Pathophysiologie sowie den klinischen Status anzeigen sowie (iii) mögliche Nebeneffekte der therapeutischen Behandlung erklären. Zudem trägt die systematische Betrachtung der Fettleibigkeit und deren Komplikationen unter Verwendungenrichedgenome-scalemetabolicmodels des Fettgewebes (iAdipocyte1850) dazu bei,die sogenannte P4-Medicine (personalized, predictive, preventive und participatory) weiter zu entwickeln.
Publikationen
Barayeu, U., M. Lange, L. Méndez, J. Arnhold, O. I. Shadyro, M. Fedorova, and J. Flemmig (2019). "Cytochrome c auto-catalyzed carbonylation in the presence of hydrogen peroxide and cardiolipins." The Journal of Biological Chemistry 294(6): 1816-1830. SysMedOs doi.org/10.1074/jbc.RA118.004110.
Bhandari, D. R., G. Coliva, M. Fedorova, and B. Spengler (2020). "Single Cell Analysis by High-Resolution Atmospheric-Pressure MALDI MS Imaging." Methods Mol Biol 2064: 103-111. SysMedOS doi.org/10.1007/978-1-4939-9831-9_8.
Brakel, A., D. Volke, C. N. Kraus, L. Otvos, and R. Hoffmann (2019). "Quantitation of a Novel Engineered Anti-infective Host Defense Peptide, ARV-1502: Pharmacokinetic Study of Different Doses in Rats and Dogs." Front Chem 7: 753. SysMedOs doi.org/10.3389/fchem.2019.00753.
Campos-Pinto, I., L. Mendez, J. Schouten, J. Wilkins, M. Fedorova, A. Pitt, P. Davis, and C. Spickett (2019). "Epitope mapping and characterization of 4-hydroxy-2-nonenal modified-human serum albumin using two different polyclonal antibodies." Free Radic Biol Med. SysMedOs doi.org/10.1016/j.freeradbiomed.2019.05.008.
Coliva, G., S. Duarte, D. Perez-Sala, and M. Fedorova (2019). "Impact of inhibition of the autophagy-lysosomal pathway on biomolecules carbonylation and proteome regulation in rat cardiac cells." Redox Biol: 101123. SysMedOS doi.org/10.1016/j.redox.2019.101123.
Colombo, S., A. Criscuolo, M. Zeller, M. Fedorova, M. R. Domingues, and P. Domingues (2019). "Analysis of oxidised and glycated aminophospholipids: Complete structural characterisation by C30 liquid chromatography-high resolution tandem mass spectrometry." Free Radic Biol Med 144: 144-155. SysMedOs doi.org/10.1016/j.freeradbiomed.2019.05.025.
Criscuolo, A., P. Nepachalovich, D. F. Garcia-del Rio, M. Lange, Z. Ni, M. Baroni, G. Cruciani, L. Goracci, M. Blüher, and M. Fedorova (2022). "Analytical and computational workflow for in-depth analysis of oxidized complex lipids in blood plasma." Nat Commun 13(6547): 1–13. SysMedOS doi.org/10.1038/s41467-022-33225-9.
Criscuolo, A., M. Zeller, K. Cook, G. Angelidou, and M. Fedorova (2019). "Rational selection of reverse phase columns for high throughput LC-MS lipidomics." Chem Phys Lipids 221: 120-127. SysMedOs doi.org/10.1016/j.chemphyslip.2019.03.006.
Criscuolo, A., M. Zeller, and M. Fedorova (2020). "Evaluation of Lipid In-Source Fragmentation on Different Orbitrap-based Mass Spectrometers." J. Am. Soc. Mass Spectrom. SysMedOs doi.org/10.1021/jasms.9b00061.
Gaud, C., B. C. Sousa, A. Nguyen, M. Fedorova, Z. Ni, V. B. O’Donnell, M. J. O. Wakelam, S. Andrews, and A. F. Lopez-Clavijo (2021). "BioPAN: a web-based tool to explore mammalian lipidome metabolic pathways on LIPID MAPS." F1000Research 10: 4. SysMedOS f1000research.com/articles/10-4/v2.
Koelmel, J. P., J. J. Aristizabal-Henao, Z. Ni, M. Fedorova, S. Kato, Y. Otoki, K. Nakagawa, E. Z. Lin, K. J. Godri Pollitt, V. Vasiliou, J. D. Guingab, T. J. Garrett, T. L. Williams, J. A. Bowden, and M. Penumetcha (2021). "A Novel Technique for Redox Lipidomics Using Mass Spectrometry: Application on Vegetable Oils Used to Fry Potatoes." J Am Soc Mass Spectrom. SysMedOS www.ncbi.nlm.nih.gov/pubmed/34096708.
Kofeler, H. C., T. O. Eichmann, R. Ahrends, J. A. Bowden, N. Danne-Rasche, E. A. Dennis, M. Fedorova, W. J. Griffiths, X. Han, J. Hartler, M. Holcapek, R. Jirasko, J. P. Koelmel, C. S. Ejsing, G. Liebisch, Z. Ni, V. B. O'Donnell, O. Quehenberger, D. Schwudke, A. Shevchenko, M. J. O. Wakelam, M. R. Wenk, D. Wolrab, and K. Ekroos (2021). "Quality control requirements for the correct annotation of lipidomics data." Nat Commun 12(1): 4771. SysMedOS www.ncbi.nlm.nih.gov/pubmed/34362906.
Kreiter, J., A. Rupprecht, L. Zimmermann, M. Moschinger, T. I. Rokitskaya, Y. N. Antonenko, L. Gille, M. Fedorova, and E. E. Pohl (2019). "Molecular Mechanisms Responsible for Pharmacological Effects of Genipin on Mitochondrial Proteins." Biophys J 117(10): 1845-1857. SysMedOS doi.org/10.1016/j.bpj.2019.10.021.
Kyle, J. E., L. Aimo, A. J. Bridge, G. Clair, M. Fedorova, J. B. Helms, M. R. Molenaar, Z. Ni, M. Oresic, D. Slenter, E. Willighagen, and B. M. Webb-Robertson (2021). "Interpreting the lipidome: bioinformatic approaches to embrace the complexity." Metabolomics 17(6): 55. SysMedOS www.ncbi.nlm.nih.gov/pubmed/34091802.
Lange, M., and M. Fedorova (2020). "Evaluation of lipid quantification accuracy using HILIC and RPLC MS on the example of NIST® SRM® 1950 metabolites in human plasma." Anal Bioanal.Chem: 1–12. SysMedOs doi.org/10.1007/s00216-020-02576-x.
Lange, M., Z. Ni, A. Criscuolo, and M. Fedorova (2018). "Liquid Chromatography Techniques in Lipidomics Research." Chromatographia. SysMedOs doi.org/10.1007/s10337-018-3656-4.
Mishima, E., J. Ito, Z. Wu, T. Nakamura, A. Wahida, S. Doll, W. Tonnus, P. Nepachalovich, E. Eggenhofer, M. Aldrovandi, B. Henkelmann, K.-i. Yamada, J. Wanninger, O. Zilka, E. Sato, R. Feederle, D. Hass, A. Maida, A. S. D. Mourão, A. Linkermann, E. K. Geissler, K. Nakagawa, T. Abe, M. Fedorova, B. Proneth, D. A. Pratt, and M. Conrad (2022). "A non-canonical vitamin K cycle is a potent ferroptosis suppressor." Nature 608: 778–783. SysMedOS doi.org/10.1038/s41586-022-05022-3.
Narzt, M. S., I. M. Nagelreiter, O. Oskolkova, V. N. Bochkov, J. Latreille, M. Fedorova, Z. Ni, F. J. Sialana, G. Lubec, M. Filzwieser, M. Laggner, M. Bilban, M. Mildner, E. Tschachler, J. Grillari, and F. Gruber (2019). "A novel role for NUPR1 in the keratinocyte stress response to UV oxidized phospholipids." Redox Biol 20: 467-482. SysMedOS doi.org/10.1016/j.redox.2018.11.006.
Ni, Z., G. Angelidou, R. Hoffmann, and M. Fedorova (2017). "LPPtiger software for lipidome-specific prediction and identification of oxidized phospholipids from LC-MS datasets." Scientific Reports 7(1): 15138. SysMedOs doi.org/10.1038/s41598-017-15363-z.
Ni, Z., G. Angelidou, M. Lange, R. Hoffmann, and M. Fedorova (2017). "LipidHunter Identifies Phospholipids by High-Throughput Processing of LC-MS and Shotgun Lipidomics Datasets." Analytical Chemistry 89(17): 8800-8807. SysMedOS doi.org/10.1021/acs.analchem.7b01126.
Ni, Z., L. Goracci, G. Cruciani, and M. Fedorova (2019). "Computational solutions in redox lipidomics - Current strategies and future perspectives." Free Radic Biol Med. SysMedOS doi.org/10.1016/j.freeradbiomed.2019.04.027.
Ni, Z., B. C. Sousa, S. Colombo, C. B. Afonso, T. Melo, A. R. Pitt, C. M. Spickett, P. Domingues, M. R. Domingues, M. Fedorova, and A. Criscuolo (2019). "Evaluation of air oxidized PAPC: A multi laboratory study by LC-MS/MS." Free Radic Biol Med. SysMedOs doi.org/10.1016/j.freeradbiomed.2019.06.013.
Sochorová, M., K. Vávrová, M. Fedorova, Z. Ni, D. Slenter, M. Kutmon, E. L. Willighagen, S. Letsiou, D. Töröcsik, M. Marchetti-Deschmann, S. Zoratto, C. Kremslehner, and F. Gruber (2022). "Research Techniques Made Simple: Lipidomic Analysis in Skin Research." J Invest Dermatol 142(1): 4–11.e11. SysMedOS doi.org/10.1016/j.jid.2021.09.017.