SP 4
Image analysis and mining for the development of predictive and prognostic models for HCC diagnosis and therapy response
The ultimate goal of this subproject is the combination of various sources of information into statistical models in order to enable a better diagnosis of HCC and predict the probability of success of a given treatment.
Initially imaging methods like MR, MET and CT will be considered as sources of information. In order to be able to correlate these in a meaningful way it is necessary to align them spatially. This means that image processing methods (image registration) have to be applied in order to correct for patient motion between different image acquisitions. In case of acquisitions performed at different time points, for example over the course of the disease, it is also necessary to compensate actual changes in the patient anatomy due to, for instance tumor growth or loss/gain of weight.
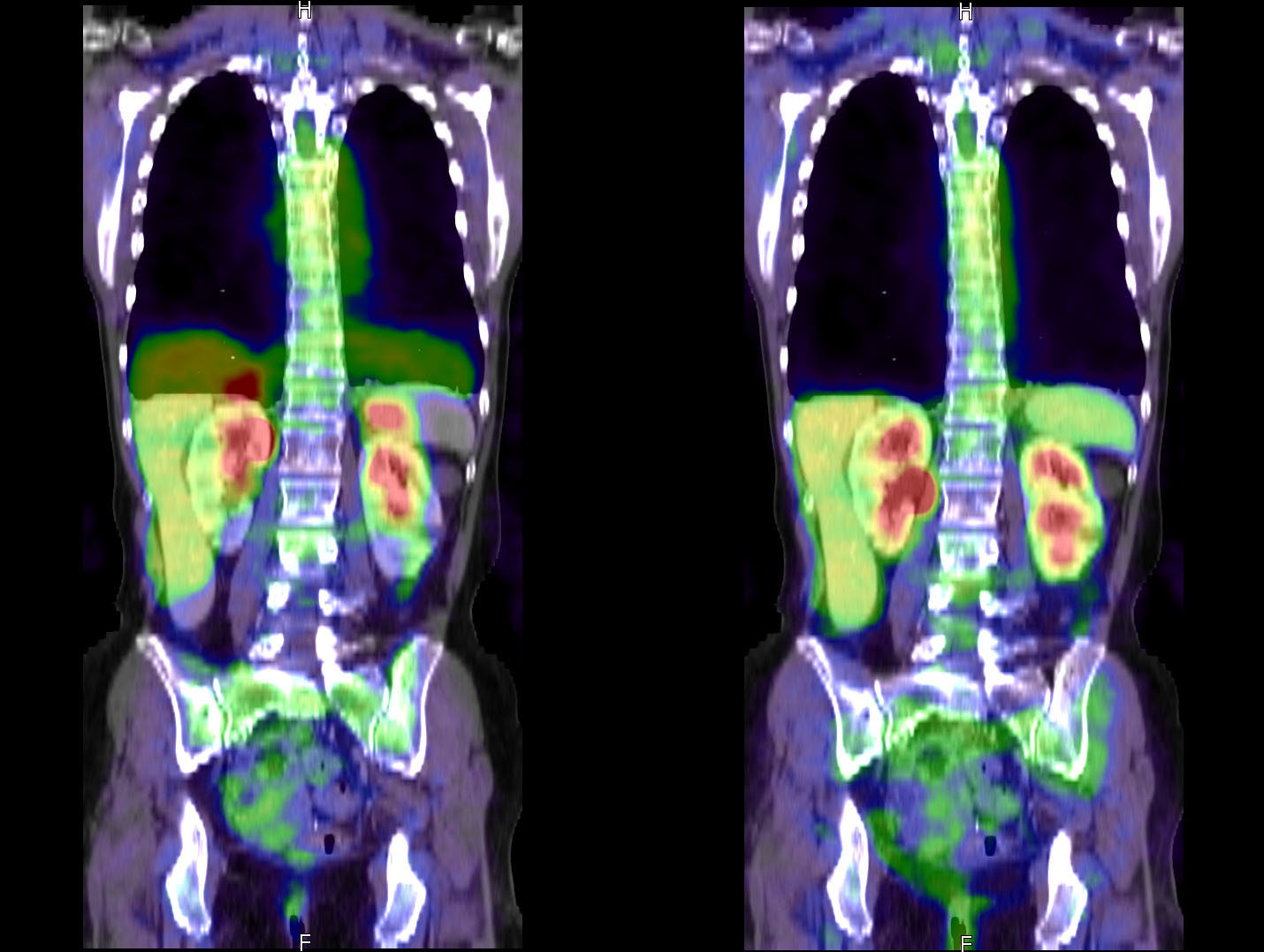

In a second step feature candidates are extracted from the image data. In simple cases these may be just the image intensities in a certain area, but more complicated features like texture based features that try to capture the local appearance of tissue (homogeneous, heterogeneous etc.) are certainly also of interest. These image based features can be combined with additional patient information such as age, sex etc. and also OMICS data (genomics, metabolomics, proteomics). To extract useful results from these multitudes of information statistical models for classification or regression will be used. For example one can train these models with patient data for which the course of the disease is already known, in order to be able to predict for new patients which course of disease is the most likely. Similar approaches are possible for the prediction of treatment success. The models can be trained with patient data that underwent a certain treatment and the results of that treatment, to predict how viable and promising the application of that treatment is for a certain new patient.
This is a very important step towards a personalized healthcare system, in which not every patient with the same disease is treated the same, but instead each patient gets a treatment tailored to his specific needs, which promises the best results possible.
Keywords: image registration, statistical models, classification, regression, data mining, diagnosis, therapy prediction