SP 2
Knowledge management and biomarker discovery
Medical research currently assumes that an increase in available data will eventually lead to improved comprehension of disease and better therapy. However, data needs to be brought into context before understanding and action can be derived from it. A data point "red" will remain meaningless until brought into context "traffic light". And even then we have to learn, often painfully, that "stop" is the appropriate action. The Biomax BioXM(TM) knowledge management environment is used in SYS-Stomach to collect current knowledge on gastric carcinoma, integrate it with the systematic experimental measurements of gastric cancer cells and structure it in a way that allows the generation of computational disease models. One of the challenges is the inconsistent way biomedical knowledge is described and deposited. Next to 21 million publications more than 1 500 specialised databases each cover some relevant knowledge. To integrate information from this diversity of resources appropriate categories and concepts such as "gene", "drug" or "human" must be identified. These allow to describe information such as "mutation (in gene) causes cancer (disease - human) in a structured way. The semantic mapping of heterogeneous information generates a gigantic knowledge network which feeds further analysis.
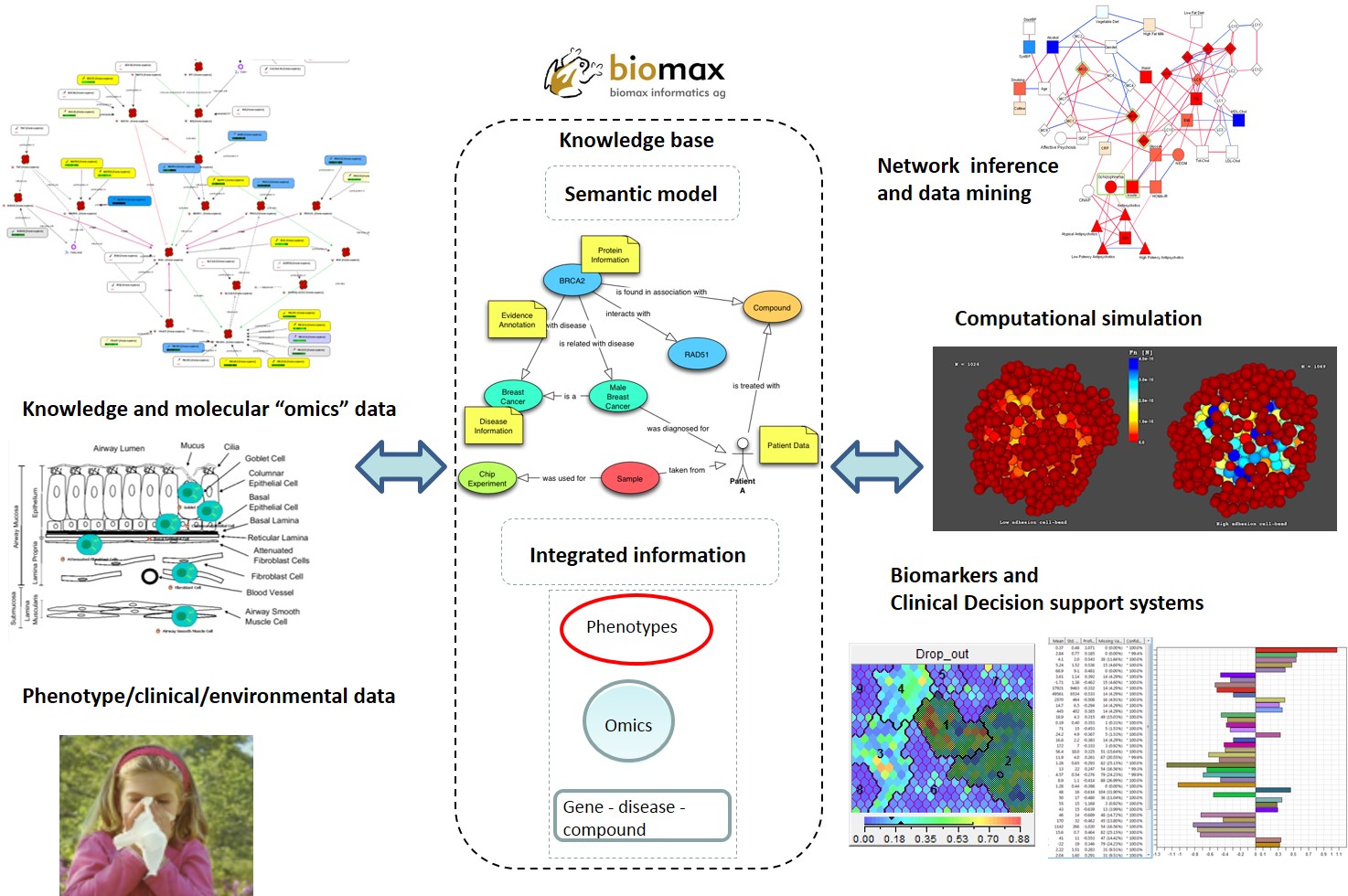
Part of this analysis is based on machine learning methods which scan large amounts of data for noticeable pattern. The Viscovery(R) data mining suite uses so called "self organising maps" which are based on the principles of neural networks. Similar to our brain, which filters our name from the background noise of a party, this method organises the data by similarity and then detects groups (clusters) of higher resemblance. Sorting these groups by criteria such as "healthy" and "diseased" allows statistical methods to filter those parameters which eventually enable predictions. Coming back to the traffic light example, the colour of the light would be important not the height of the pole.
Keywords: Knowledge management, machine learning, semantic mapping, network analysis